Building a 360° View of the Patient Experience by Using a Unified Clinical Data Management Platform
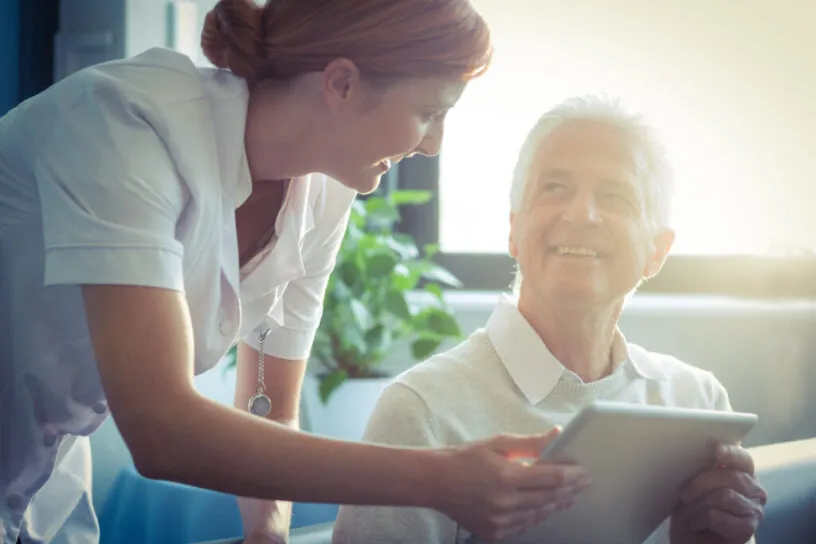
Clinical trials have evolved rapidly over recent years. They now have highly sophisticated protocols and multiple divergent data sources, inordinate data volume, decentralization, and increasingly complex adaptive designs. These changes have led to significant clinical data management challenges because the data management technologies and processes have not progressed at the same breakneck speed.
Clinical data management solutions are often built with a mix of disparate tools that are homegrown or from different vendors, so the individual components lack interoperability. This leads to disconnected silos with duplicative and inconsistent data that are challenging to reconcile, ultimately making it very difficult to produce holistic views of the patient experience.
This is why an increasing number of sponsors and clinical research organizations (CROs) are tapping into unified, intelligent, and secure modern clinical data management solutions. These solutions merge different data streams—including both subjective (e.g., PROs -Patient Reported Outcomes) and objective data (e.g., wearables/sensors)—through a common model so that you can easily and quickly visualize and analyze clinical trial data in a unified way. They also reduce the risk to data integrity, quality, and patient safety. Since many data streams are complementary, you can develop aggregated views of patient data that are hugely informative for how patients are affected by drugs and diseases.
Some of the key features that are making it possible to develop more comprehensive views of patient data include the following:
Agility: Study designs must be more data acquisition focused, regardless of source across all data assets. Data capture and clinical data management processes must be designed with agility in mind to enable changes in data capture to meet the custom needs of an individual study, site, or patient (e.g., ePRO/eCOA, eConsent, sensors/wearables). It is important to define the full schedule of assessments (visits), the set of protocol activities, and any streaming or other study aspects that may not be visit related. This will also include other characteristics, including cohort, arm, and study phase design, that provide context and drive behavior during execution.
Common Data Model: The model must support a wide variety of data from a diverse group of sources and co-localize the disparate data for algorithmic cleaning, AI-assisted workflows, and custom data extractions and models. For instance, whether “systolic blood pressure” is designated as SBP or Sys BP does NOT drive the meaning of the data point. The semantic meaning, which is systolic blood pressure, is the key. A common data model allows drug and device developers to focus on the implications of their analyses rather than wasting time and resources on the nuances of ingesting and harmonizing disparate clinical datasets.
Scalable: Data capture must be scalable for all types and volumes, ranging from simple laboratory test results to high-volume, high-velocity data from medical-grade sensors/wearables. Scalability is also achieved by automating data review with machine learning and displaying downstream outcomes in easily interpreted visualizations based on a single source of truth that allows all program and study stakeholders to consume the data’s meaning.
Secure: When asked to rate EDC product attributes by their impact on clinical trial success, respondents to an Industry Standard Research survey selected data security as the attribute with the overall highest impact, with 48% and 34% saying it has high or moderate impact, respectively (Industry Standard Research, 2020). Therefore, you should seek a partner that has a solid track record of data security and acting as a custodian of patient trust. They should have ISO 27701:2019 certification, which is the closest to a General Data Protection Regulation certification that can be obtained, and SOC 2 Type 2 “Plus,” which has additional controls over and above the basic AICPA professional standards for both the Information Security and Privacy trust principles.
Example Scenario: Holistic Approach to Patient Data Capture and Monitoring
In a clinical trial conducted to test a new type of diuretic in patients with congestive heart failure, a holistic approach to patient data capture and monitoring helped avert negative patient outcomes. The trial involved remotely collecting data using various sources, including a biosensor patch, a smart weight scale, smart urine strips, eConsent, and ePRO.
Since the site was able to view both the sensor and ePRO data, they were able to quickly detect data anomalies that they could then act on, such as determining if patients needed to be checked on by a nurse via video visit and whether the patients should go to a hospital for additional tests. By using this comprehensive approach, negative patient outcomes were averted due to real-time data observed by multiple stakeholders (site, sponsor, and CRO), including the ability to review sensor and ePRO data together, and withdrawing patients if necessary.
Summary
As clinical trials continue to modernize, you will increasingly face new and changing clinical data management challenges that will only worsen if your clinical data management technologies and processes are not unified, intelligent, automated, and secure. By deploying modern solutions that can merge different clinical trial data streams through a common model, you can easily and quickly visualize and analyze your data in a unified way, building a truly comprehensive view of the patient experience.
Download our white paper to learn how to succeed in an increasingly complex clinical trial world:
Contact Us
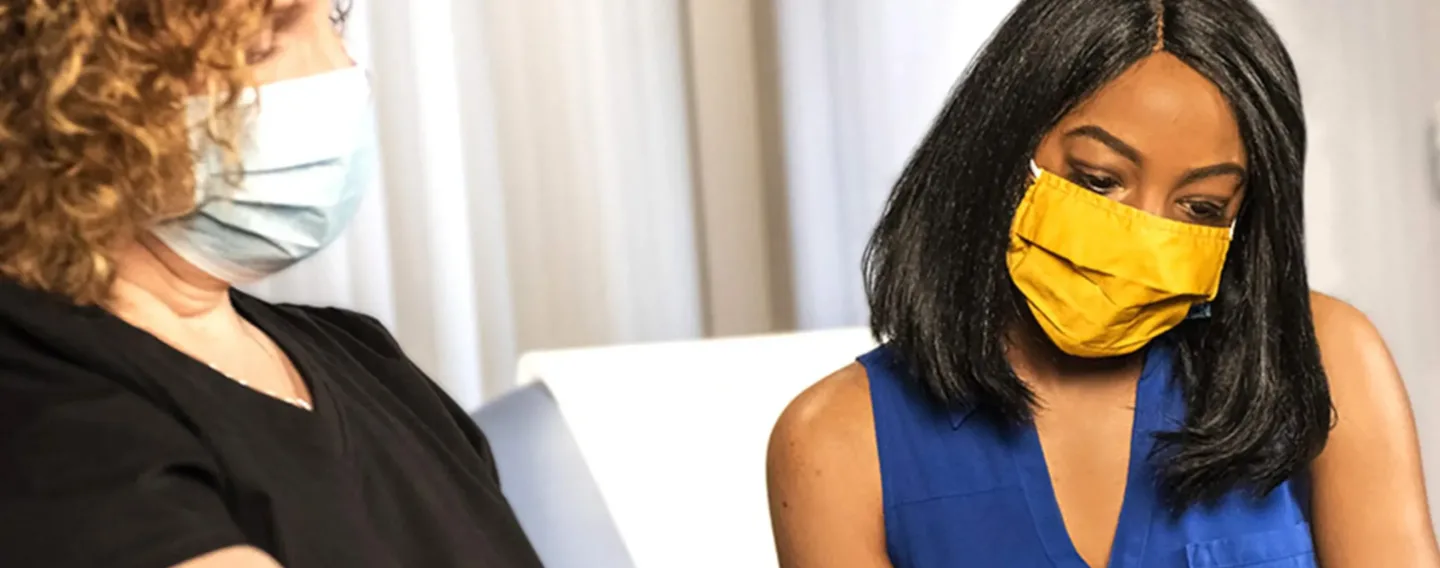