Transforming Medical Coding and Data Review by Harnessing AI/ML and Automation
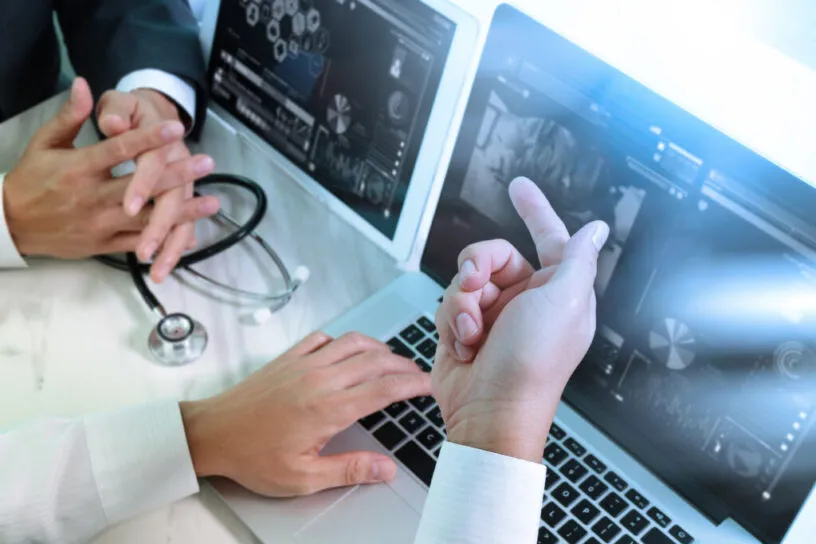
This blog was authored by Freddy Mendieta, Director, Product Management, Coder/Coder+/Safety Gateway at Medidata.
Life sciences companies are seeking ways to harness new technologies like AI/ML. There’s a mix of emotions ranging from excitement to hesitation about using these technologies. Let’s explore some of the challenges and benefits—while keeping in mind the goal of helping customers to be more efficient so they can focus on what matters most.
Accurate Medical Coding Is the Foundation of Clinical Research
The importance of accurate coding in clinical research cannot be overstated. First, it ensures patient safety by enabling the timely identification and evaluation of certain data sets, such as adverse events (AEs). For instance, coding errors can lead to missed or delayed detection of safety signals, preventing appropriate action that can protect patients and potentially jeopardizing clinical trial efficacy.
Accurate coding also underpins the success of many other key clinical research functions, including other analyses (e.g., concomitant medications), risk-benefit assessments, and regulatory compliance. It also improves study comparability, enhances publication quality, addresses legal and liability concerns, and promotes overall efficiency in resource utilization.
Coding Challenges Associated with Standardized Dictionaries
Standardized medical coding dictionaries for clinical trials—such as WHODrug and MedDRA— assign standard codes for concepts or conditions including AEs, medical history, procedures, and concomitant medication, among others. These resources contain a vast number of terms covering a wide range of medical conditions, drug-related concepts, and AEs. Understanding and correctly applying these terms requires a strong knowledge of medical terminology.
Different medical terms can describe the same concept or condition, leading to challenges in choosing the most appropriate terms. Variations in language, cultural differences, and diverse medical practices across regions should also be considered.
Coding dictionaries are regularly updated to include new terms and concepts. Keeping up with these evolving standards and ensuring consistent coding can also be challenging as manually coding large volumes of medical data is time-consuming and resource-intensive.
AI/ML Adoption Barriers
Several barriers can impede sponsors from adopting AI/ML technologies in clinical trials. A primary concern is the data-intensive nature of AI/ML model generation, which can result in models that are too broad and lack specificity. Smaller sponsors often require models tailored to their specific therapeutic areas and needs, while larger sponsors may be more comfortable with generic models.
Regulatory hurdles also contribute to hesitation in AI/ML adoption, especially concerns around data privacy and the use of AI/ML algorithms. But regulators worldwide have been steadily issuing guidelines and regulations to facilitate the adoption of these technologies in a responsible and compliant manner.
Integrating AI/ML into the medical coding process requires change. This can be challenging for sponsors accustomed to established methods (i.e., the “old is gold” view) since updating SOPs, policies, and training can require significant investments in cost, time, and effort.
Lastly, some sponsors express apprehension about the novelty of AI/ML and data quality (i.e., "garbage in, garbage out"). But these concerns are largely unfounded since manual review remains a key component of the coding process. This human oversight ensures that sponsors can maintain high confidence in the validity of the data output, despite the integration of AI/ML technologies.
Future Scope of AI/ML in Coding
Looking beyond these barriers, AI/ML is positioned to enhance the coding process significantly. One key advantage is its potential to eliminate coder bias—both conscious and unconscious—since it analyzes data directly. By reducing manual effort, resources can be redirected toward other priorities, such as reviewing verbatims and updating SOPs, policies, and training protocols.
As noted earlier, consistency is a major challenge in medical coding that can be greatly improved through AI/ML. These technologies can reduce inconsistencies by flagging coding differences as they occur during or after trials. Future developments may even enable AI/ML systems to categorize these discrepancies as 'low' or 'high' risk, so medical coders can focus on data points that matter most.
By leveraging historical knowledge, AI/ML can provide more concrete coding choices. While coding dictionaries are complex and have many options, AI/ML can narrow down these options based on historical coding practices. This can reduce the amount of time coders spend on research and manual intervention. As these technologies gain wider adoption and more training data becomes available, customized AI/ML models can be developed to cater to specific companies, regions, therapeutic areas, or study phases.
Finally, as AI/ML technologies continue to advance, they have the potential to streamline coding processes further. Improved algorithms should increase autocoding hit rates, potentially eliminating synonym lists. As models learn from the review process, the number of items requiring manual approval by coding specialists will decrease. Overall, fewer manual processes result in more time for resource planning and focusing on areas that help ensure data accuracy.
The Evolution of Automated Medical Coding Workflows for Rave EDC.
Medidata Rave Coder+ is a tool that provides medical coding for clinical trials in English and Chinese using WHODrug and MedDRA dictionaries. Rave Coder+ is built on the Medidata Platform with a connected, automated medical coding workflow for coding verbatim terms entered in Rave EDC.
Freddy Mendieta is the product management lead for Medidata's Rave Coder and Coder+ medical coding solutions. He’s a product management executive with a track record of delivering solutions to clients that meet and exceed their expectations. Freddy is an experienced leader who leads global teams to deliver solutions the market will adapt and leverage.
Contact Us
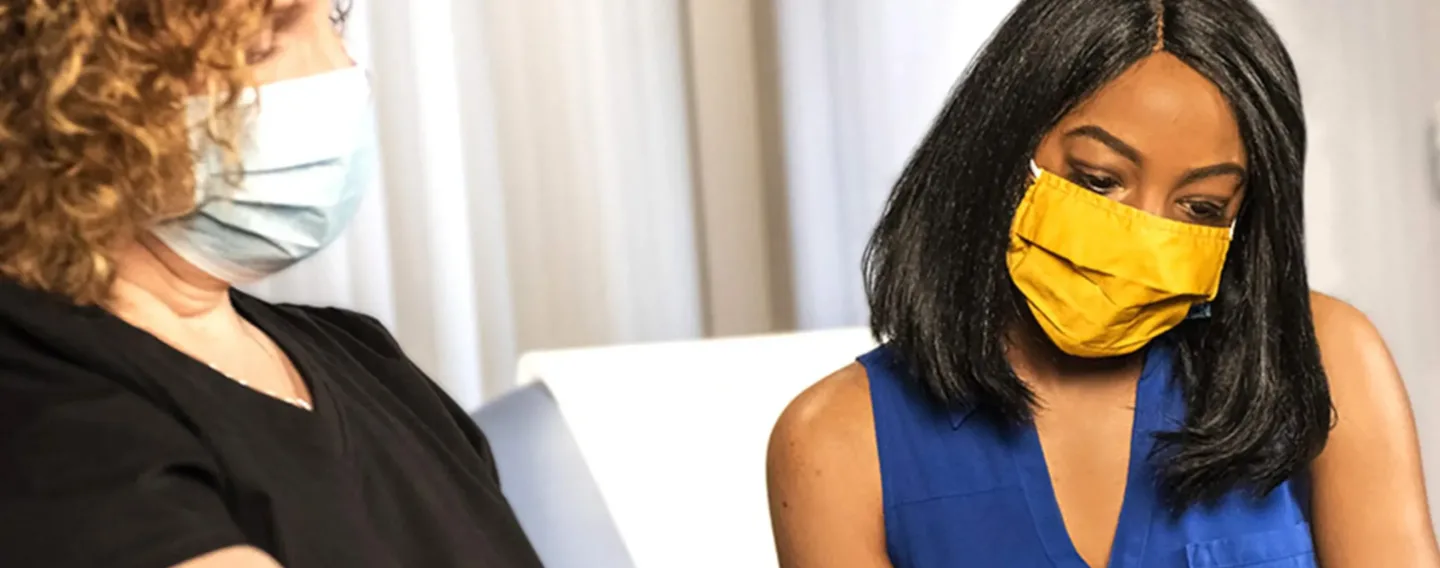