The Role of AI and Big Data in Clinical Trial Evidence Generation
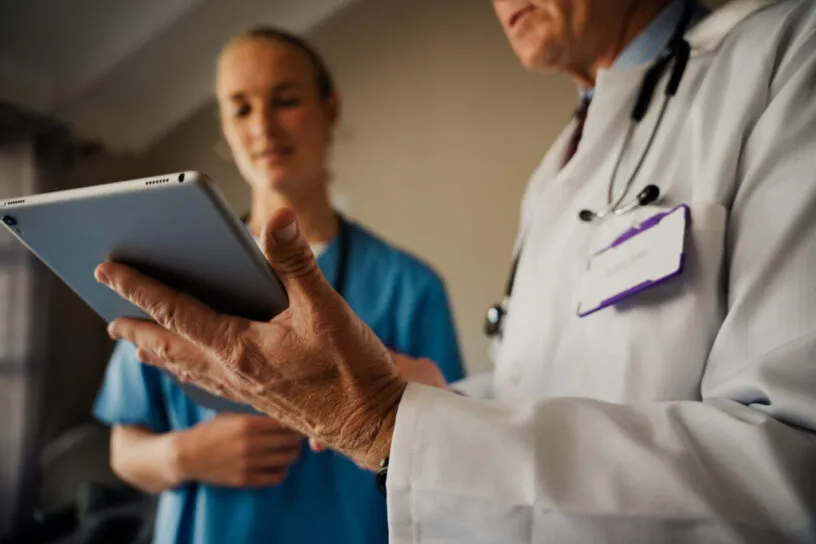
Arnaub Chatterjee, SVP of Medidata AI sits down with MIT Technology Review to Discuss the Future of Evidence Generation.
Artificial intelligence and data can help fuel new ways of working on clinical trials and beyond. AI and advanced analytics technology can reduce uncertainty in trial planning for sponsors and CROs—meaning a faster time to market, reduced risks, lowered costs, and fewer burdens on patients.
In the second installment of this three-part blog series, Arnaub Chatterjee, SVP of Medidata AI, joined MIT Technology Review’s Business Lab podcast to answer key questions about how AI technologies will impact clinical trial evidence generation.
How has data and evidence generation evolved in the last couple years?
Ten years ago, the term “real-world data,” or RWD, didn’t even exist. What we’re seeing now is that regulatory agencies—through very important pieces of legislation like the 21st Century Cures Act—have jump-started how RWD can be used to augment our understanding of treatments and of disease.1
Now that AI-enabled drug development is gaining momentum, the challenge becomes: how do we keep the rigor of the clinical trial and tell the entire story while also bringing in RWD to complete that picture?
It’s a problem we’ve been focusing on for the last two years at Medidata. During COVID, we built Medidata Link, a solution that ties together patient-level clinical trial data and RWD. Initially, we started work on this product with a COVID vaccine manufacturer to help them see if their vaccine was going to be safe and efficacious over time. This type of long-term study is going to play a big part in how clinical trial data collection evolves in the future.
What are some other gaps and challenges that you have seen in generating high-quality evidence?
The elephant in the room is that despite all of the advancements in data and analytics, the probability of technical or regulatory success is still really low. The overall likelihood of approval from phase one consistently sits under 10% for a number of different therapeutic areas.2 What underlies these failures is a lack of clinical trial data to demonstrate efficacy. In many cases, companies submit or include what the regulatory bodies call a flawed study design or an inappropriate statistical endpoint. These trials may also be underpowered, meaning the sample size was too small to reject the null hypothesis.
When designing a clinical trial, there are so many questions to keep in mind: what are my primary and secondary endpoints? What inclusion or exclusion criteria do I select? What’s my comparator? How do I understand outcomes? How do I understand the mechanism of action? There are a myriad of different choices and decisions that have to be made in parallel—relying on mostly RWD. While RWD can fill some gaps, it leaves sponsors with even more questions to answer: how is the data collected? How do you verify where it came from? Can it be trusted?
At Medidata, we’ve been trying to navigate how to use RWD in a robust way while accounting for these critical issues. Our approach is to use a curated historical clinical trial dataset of 28,000+ clinical trials that sits on our platform to contextualize RWD. We’ve been seeing that this approach helps clinical development teams better understand how patients are responding to therapy and design data-driven trial protocols to improve statistical analysis.
How are companies actually leveraging this novel type of data to build faster, more effective treatments?
At Medidata, we help companies leverage this novel data in three different areas. One path is to use historical clinical trial data throughout the entire development process to make sharper decisions, avoid protocol amendments that add six to 12 months to trial timelines, and generate high-quality, regulatory-grade output. Another path customers can take is using a Synthetic Control Arm®—leveraging historical clinical trial data to augment or replace a traditional control arm in areas like rare disease or in single-arm trials. Our third path is using Medidata Link to bridge data between clinical trials and the real world.
For example, we work with many companies in the chimeric antigen receptor T-cell therapy, or CAR-T, space—an area of clinical research that is often plagued with safety issues. In CAR-T, we use our historical clinical trial dataset to create machine learning algorithms that predict when serious adverse events will occur. Recently, we’ve built models alongside the Cleveland Clinic that were ~90% accurate at predicting cytokine release syndrome, one of the most serious adverse events in CAR-T therapy that contribute to patient death.3 This data opens up many possibilities that we’re exploring at Medidata, like how to use AI to screen trial patients who are prone to an adverse event or, even more importantly, how to prevent an unnecessary death or adverse event in future trials.
Listen to the full discussion here.
Contact Us
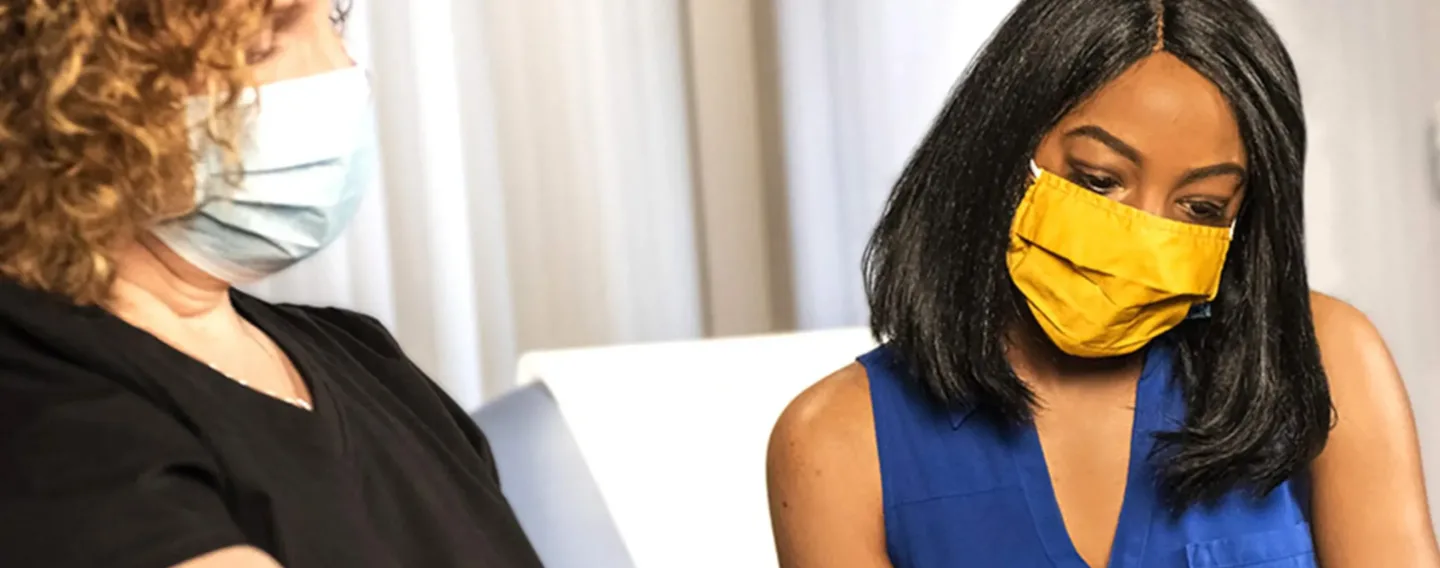