Medidata Blog
AI Technology: The Future of Advanced Analytics in Clinical Trials
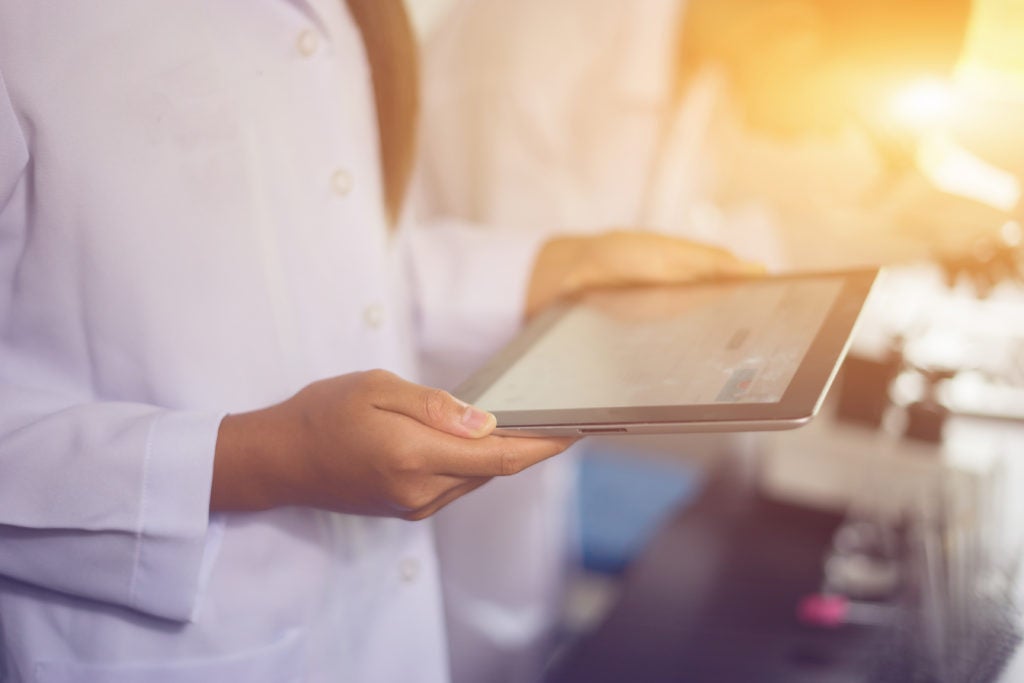
Over the last few decades, the stream of data available to life sciences companies has grown from a trickle to a tidal wave: genetic and genomic portraits of individual patients, metabolomic and proteomic profiles, real-world data from wearables measuring everything from heart rate variability to blood glucose levels, and detailed patient clinical histories from electronic health records. Today, approximately 30% of the world’s data volume is being generated by the healthcare industry. By 2025, the compound annual growth rate of data for healthcare will reach 36%. That’s 6% faster than manufacturing, 10% faster than financial services, and 11% faster than media & entertainment. Furthermore, the quantity of patient data housed in clinical systems grew nearly 500% from 2016 to 2020.
Data analysis has flourished, too. Alongside classical statistics, powerful artificial intelligence (AI) technologies have emerged that can manipulate massive numbers of inputs and curate data stored in non-standard formats. One branch of AI called machine learning can identify patterns in data without any starting hypotheses—which means humans don’t need to make prior assumptions about what surprises might be lurking there.
The new AI tools, combined with the boom in healthcare data, will transform clinical trials and drug discovery. Morgan Stanley Research believes that the use of artificial intelligence and machine learning could lead to an additional 50 novel therapies over a 10-year period, which could translate to a more than a $50 billion market. Researchers are already using machine learning tools in combination with statistical analysis to uncover new insights from vast repositories of real-world data and clinical histories.
For example, Medidata has used big data modeling techniques to find lab markers that can predict a chimeric antigen receptor T-cell therapy (CAR T) patient’s chance of developing severe cytokine release syndrome (CRS). Previous investigations of the clinical risk factors of severe CRS relied on very small patient populations usually drawn from a single CAR T study. Typically, a CAR T study averages only 11 patients. Instead, Medidata used a large, pooled clinical trial data set spanning 540+ patients from multiple CAR T clinical trials to link CRS risk to common biomarkers.
Life sciences companies are also beginning to use artificial intelligence technology to ensure clinical trials produce regulatory quality data, sorting and classifying data entry errors, outliers, inconsistencies, and misreported adverse events to speed up the drug approval process.
And yet, most life sciences companies still aren’t using AI tools and machine learning technology for clinical data analysis to their fullest potential. That’s partly because AI is new, and partly because the probability of technical or regulatory success is still very low despite these advancements. But it may also reflect a lack of understanding about what AI technology can do and how it differs from statistics.
One succinct way to describe the distinction between the two: statistics accomplishes what is hard for humans and easy for computers, whereas artificial intelligence tackles things that are hard for computers and easy for humans. The former spits out p-values, while the latter struggles with speech recognition and image recognition. One field of study, known as machine learning, combines AI with statistics to tackle the things that are hard for both computers and humans.
What is Statistics?
Classical statistical modeling techniques were developed between the 18th and early 20th centuries to study, quantify, and describe populations, economies, and moral actions. But they were generally adapted to much smaller datasets than those currently available. The discipline exploded in popularity in the 1980s with the emergence of Bayesian modeling, which allows statisticians to estimate probabilities.
Statistical modeling became essential to drug development after amendments took effect in 1962 that required any drugs approved for the market to show proof of efficacy. Today, statistics is commonly used to evaluate how much better a therapy works than a placebo or standard of care to treat a patient population.
Statistics is designed to make inferences about the relationship between variables—to determine the input variable’s impact on the output variable. But it’s less suited for large data sets with vast amounts of input data where the relationship between variables is unknown. It becomes cumbersome and unwieldy to evaluate the statistical significance of each input variable. Statistical modeling requires the statistician to develop tight assumptions about the problem or question being analyzed—especially data distributions—before the models are run.
What Is Artificial Intelligence?
Although artificial intelligence has become something of a buzzword in the past decade, it dates to the invention of modern computing, so it’s no newcomer to the field of analytical modeling. AI technology aims to understand human intelligence—particularly human skills such as recognizing objects and sounds, speaking, translating, and performing social transactions or creative work—in order to replicate this intelligence in machines.
In life sciences, AI can be taught to differentiate cancer cells in a laboratory, to identify patterns in high quality medical images such as x rays, and to analyze complex sets of genomic data. AI analytics also rapidly combine consumer data, treatment data, diagnoses, lab tests, and other information stored in natural language to identify unexpected or novel patterns and to predict treatment responses and patient behavior.
What Is Machine Learning?
Machine learning is a subfield of computer science and artificial intelligence that aims to build systems that can learn from data, rather than just follow explicitly programmed instructions. Machine learning was made possible by cheap computing power and the availability of massive amounts of data from which computers could “learn.”
Machine learning is built on a foundation of statistical inference, but it does not require preset assumptions; this allows computers to discover insights and make classifications that human analysts can’t anticipate and to generate predictions with superhuman accuracy.
There are several types of machine learning, including supervised machine learning, unsupervised learning, and reinforcement learning. With supervised machine learning, the computer is fed data that includes the answer to the problem posed by the data set. It is used to teach the computer to make predictions about future data sets. With unsupervised learning, no output or answer data is included initially, but the algorithm makes decisions about patterns it finds in the data. Reinforcement learning, inspired by behavioral psychology, involves providing rewards and punishments to the computer to teach it to achieve a certain objective.
Unsupervised learning might take the form of processing omics data to generate relevant clusters, or associations in the data. For data quality applications, it could aid in association mapping—looking at an entire database, in an unassisted way, and identifying the relationships between two data points. This could identify unanticipated inconsistencies in a data set that otherwise cause compliance problems.
With clinical trial data volumes increasing at an exponential rate, it’s becoming increasingly difficult for life sciences companies to keep up. Machine learning algorithms can help companies analyze data and decide which pieces of information are relevant, helping draw insights from massive data volumes. Expect to see a combination of statistics and machine learning powering clinical trials of the future.
Medidata AI
Medidata AI provides unparalleled clinical data, advanced analytics, and industry expertise for pharmaceutical, biotech, and medical device leaders, to help reimagine what’s possible, uncover breakthrough insights, make confident decisions, and pursue continuous innovation. Our suite of solutions is backed by an integrated team of scientists, physicians, technologists, and ex-regulatory officials who bring deep expertise to answer your most important questions.
Medidata AI is built upon Medidata’s core platform comprising 28,000+ trials and 8.5 million patients. What makes Medidata AI unique is that our patient-level data is pulled directly from all case report forms from trials. We capture 100+ individual-level clinical fields and 35+ operational covariates.
Download our case study collection to discover how Medidata AI powers success for top biopharma organizations: