The Essential 5 Es for Operationalizing AI in Clinical Data Management
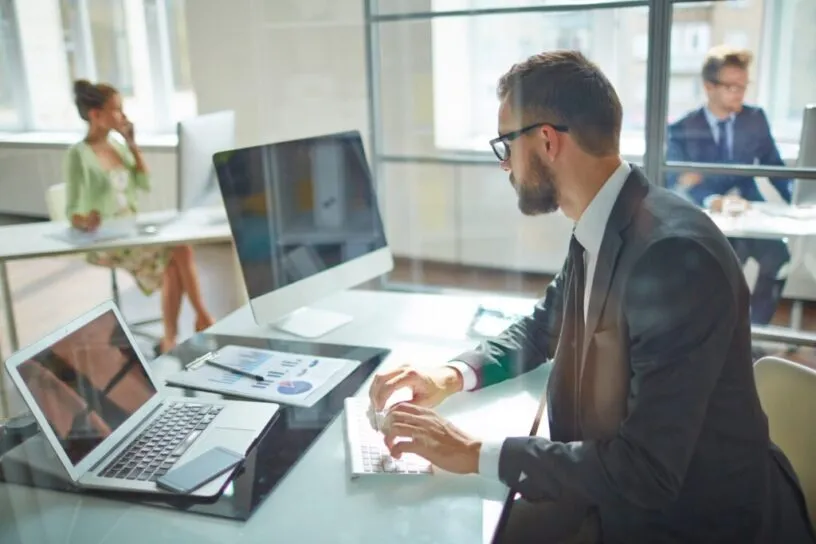
Modern clinical trials are accelerating towards increased complexity, with their expanding array of digitized source data coming from a wider variety of sources, decentralized clinical trials (DCT), and adaptive designs. Artificial intelligence (AI) is being used in many innovative applications across the entire drug and device development continuum. It can be broadly categorized into several dimensions, including machine learning (ML) systems that leverage AI to perform well-defined tasks and uncover hidden data patterns, and generative AI—the most visible form in recent years, which involves algorithms with well-defined training yet nearly limitless application capabilities.
AI holds significant potential for streamlining clinical data management (CDM) processes and optimizing the use of time and resources. At Medidata, the primary focus is on harnessing AI/ML to improve the entire clinical development lifecycle, including designing optimal clinical trials, generating evidence to support regulatory milestones, and efficiently managing clinical data.
Despite its huge potential, successful AI integration requires careful consideration of some guiding principles which we’ve encapsulated in the concept of the 5 Es: essential, efficient, enjoyable, explainable, and ethical. These principles provide a comprehensive framework for leveraging AI effectively and are further detailed below.
Essential: Does the Workflow Need AI or Simple Automation?
AI should be applied where it truly brings value, addressing problems that cannot be solved using standard data management approaches or simpler automation methods. For example, many data review checks can be automated using low/no-code environments and repeatable templates adaptable to specific study nuances, such as variable naming. These simpler methods often provide the exact outputs needed without the extensive data required to train AI algorithms. However, AI becomes essential in more complex scenarios, like ensuring consistency and completeness of safety data, or the ability to extract and interrogate large amounts of audit trail data. In these cases, AI can significantly reduce manual effort by assisting in identifying missing information, flagging inconsistencies, and presenting users only information that needs action.
Enjoyable: Can Users’ Feedback Fine-tune Output to Reduce False Positives?
The enjoyable aspect of AI in CDM refers to the holistic user experience. An AI solution should significantly improve the user experience by functioning as a virtual assistant that offers actionable recommendations. This involves reducing noise and false positives, making the system easy to use, and incorporating human-in-the-loop (HITL) mechanisms where users can provide feedback to refine outputs over time.
Additionally, all relevant information and references should be accessible within the same environment so that users can seamlessly cross-check AI outputs with underlying data. By connecting AI checks to underlying data and required actions, a data manager’s workflow can be streamlined, and their experience can be enjoyable by empowering them to focus on higher-level tasks while interacting easily with AI-generated insights.
Efficient: Will AI Enhance Workflow Integration, Create Measurable ROI, and Reduce User Burden?
It’s important to assess whether AI will bring significant efficiencies to users. For example, inadequate data for training AI algorithms or highly nuanced, protocol-specific tasks may require significant development investment, lower replicability, and result in a high number of false positives, which would diminish the return on investment (ROI). It’s also essential to consider whether existing processes are already automated and if the improvement provided by AI is substantial enough to justify additional incremental development efforts.
High-volume, repetitive tasks such as manually merging data from different sources or medical coding are prime candidates for AI applications. These tasks are high-volume, time-consuming, and can require specialized knowledge. By leveraging AI, these processes can be streamlined, allowing data managers to focus on higher-level tasks, quality improvements, and more proactive root cause analysis. Overall, leveraging AI as a virtual assistant can significantly reduce manual effort, reduce user burden, and create a significant ROI.
Explainable: Does the AI Model’s Decision-making Logic Reassure Users?
A major concern with AI is its "black box" nature, where the decision-making process can lack transparency. An explainable AI system demystifies the algorithms, making them comprehensible to all stakeholders, including data managers and regulatory bodies. This means explaining how the AI was trained, how it operates, and how it arrives at its conclusions. Explainability is important not only for user adoption but also plays into the enjoyable aspect of AI.
As an example, explaining to users that AI algorithms, such as those used in medical coding, may not always achieve 100% accuracy but can reach high levels like 92% to 94% is important. Or the ability to see confirmatory SQL statements to ensure that the dataset generated through GenAI audit trail feature is accurate. Users need to understand that ongoing human feedback is necessary to continually improve the system. By incorporating these elements, explainability ensures that users can trust and effectively interact with AI, ultimately leading to better adoption and more accurate outcomes.
Ethical: Is the AI System Designed to Prevent Bias and Ensure Fairness?
When it comes to ethics in AI systems, several key considerations must be addressed, starting with data that is used to train algorithms. Adherence to data privacy regulations is paramount, alongside evaluating whether the data could introduce biases into the algorithms. Additionally, the methodology must be robust enough to incorporate human feedback without introducing bias. This involves ensuring that feedback is aggregated and statistically significant before being applied to the algorithm, preventing any single user’s input from disproportionately affecting the outcomes.
As discussed earlier, transparency around the "black box" nature of AI is also important. Users and stakeholders need to understand how the algorithms were developed, the data used, and the processes in place to protect privacy. It’s essential to make sure that feedback data doesn’t leak between customers, maintaining strict data privacy throughout the conduct and execution of AI-powered features.
Addressing these ethical considerations ensures adherence to the highest standards of conduct for the use of data and AI. This approach not only builds trust among users and stakeholders but also aligns with regulatory requirements and promotes the responsible use of AI. By integrating ethical principles, a framework is created that supports the fair, transparent, and responsible application of AI in CDM.
Conclusion
The 5 Es—essential, efficient, explainable, ethical, and enjoyable—serve as guiding principles for the successful implementation of AI in modern data management organizations. By focusing on these key elements, AI can significantly enhance efficiency, accuracy, and the user experience, while maintaining transparency and ethical integrity.
Watch this insightful on-demand webinar for a deep dive into the 5 Es.
Contact Us
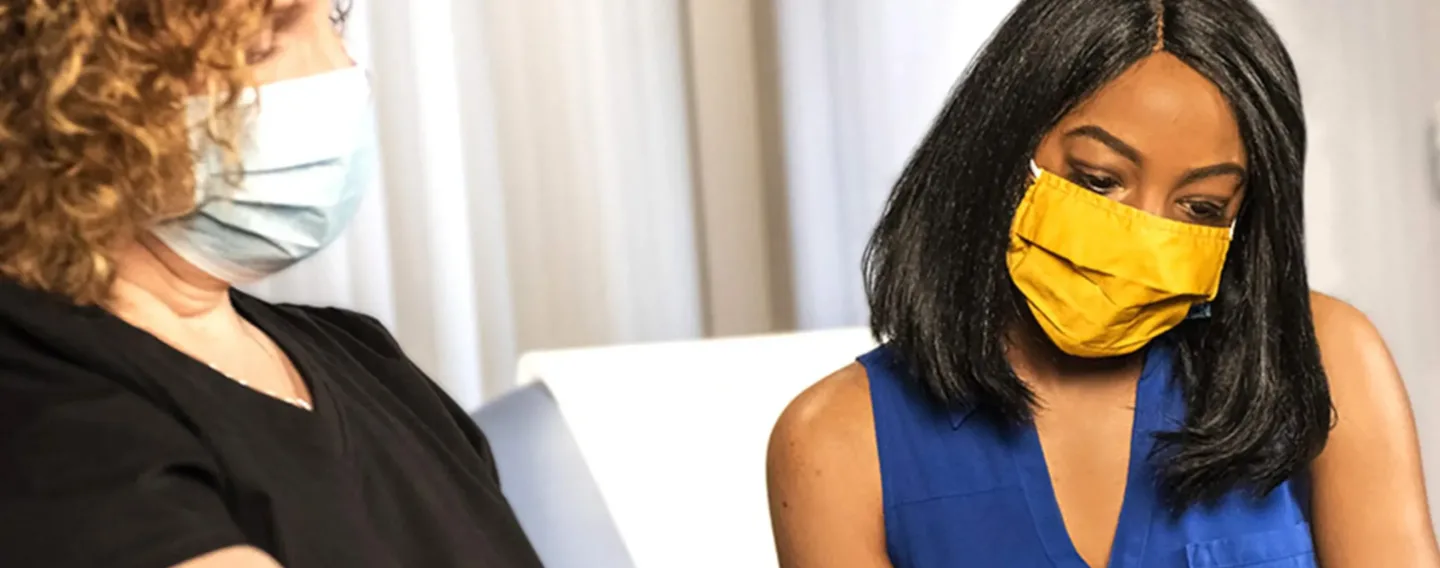