The Challenge, the Change, the Solution: Reinventing Medical Imaging
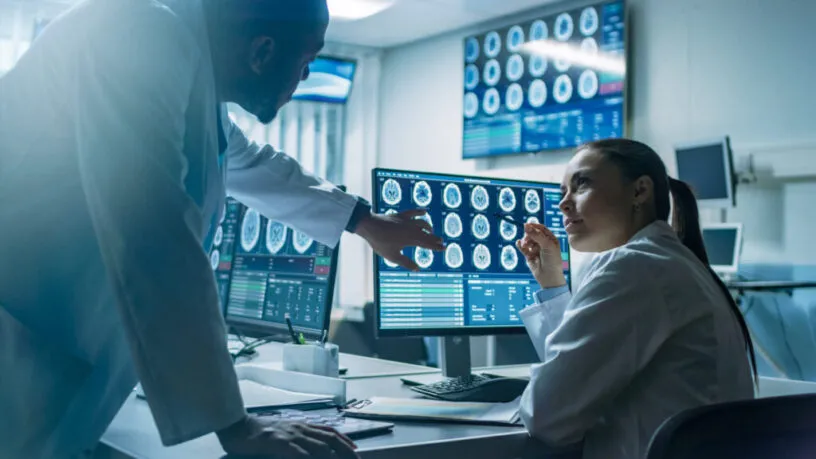
In oncology clinical trials, accurate and reliable imaging is pivotal in assessing treatment response and disease progression. Computed tomography (CT) scans, in particular, are widely used for their ability to provide detailed cross-sectional images of the body. But the value of CT imaging is only as good as its quality. Ensuring the quality of images is essential to maintain consistency, reduce variability, and ensure the credibility of trial outcomes.
The Importance of Quality Control in Medical Imaging
Clinical trials often involve multiple research sites, each with different imaging equipment, standard of care protocols, and personnel. This variability can introduce inconsistencies in image quality, which may impact the ability to make accurate and comparable assessments. Quality control (QC) processes standardize image acquisition protocols, ensuring consistency across sites.
Response Evaluation Criteria in Solid Tumors (RECIST) guidelines are commonly used to measure tumor size changes. Differences in image quality can impact the assessment of tumors, which in turn can impact trial endpoints, costs, and timelines. Examples include:
- presence and type of contrast enhancement
- anatomical coverage
- presence of artifacts
- proper field of view
- whether or not baseline and follow-up images even belong to the same patient
Robust QC processes help ensure that tumor assessments are accurate and reproducible, which is critical for determining treatment efficacy. Lacking a robust QC process can raise questions about data integrity and reproducibility with regulatory agencies and potentially impact regulatory approval. Having a thorough QC process helps mitigate these risks. Technology can play a big part in solving these QC challenges.
The Challenge
Managing and reviewing large amounts of imaging data from multiple sites is manual, time-consuming, and expensive. To save on costs, imaging QC is typically performed by an imaging technician or trained imaging specialist rather than a radiologist, which can introduce variability in QC results.
The Technology Solution
AI algorithms can automatically assess anatomical coverage—and whether baseline and follow-up images belong to the same patient—and present those results to end users. These algorithms can also perform other checks, including verifying the presence of axial images and determining if image gaps exist. This means quality results can be obtained without users having to open and review every image provided for the trial, which saves time, reduces costs, and improves variability. Results can also be presented in a way that lets users verify and modify results if needed.
The algorithms were developed by Dassault Systèmes BIOVIA group, leveraging Pipeline Pilot, a state-of-the-art data pipelining solution. And Medidata has integrated these algorithms into Rave Imaging. This collaboration demonstrates the strength of combining Medidata’s life sciences expertise with Dassault Systèmes teams and products. These two algorithms are the first of several, and eventually, all oncology quality checks will be automated and integrated with Rave Imaging. This includes the presence of intravenous contrast and proper field of view, to name a few.
Conclusion
Strong quality control of CT images is a non-negotiable aspect of oncology clinical trials. A robust QC program ensures that imaging data is accurate, reliable, and fit for regulatory submission, supporting the success of oncology trials. With the evolution of artificial intelligence and its potential to improve clinical trial procedures, gone are the days when each image had to be opened and manually reviewed for quality. This saves costs, speeds up timelines, and improves the overall quality of trial results.
Contact Us
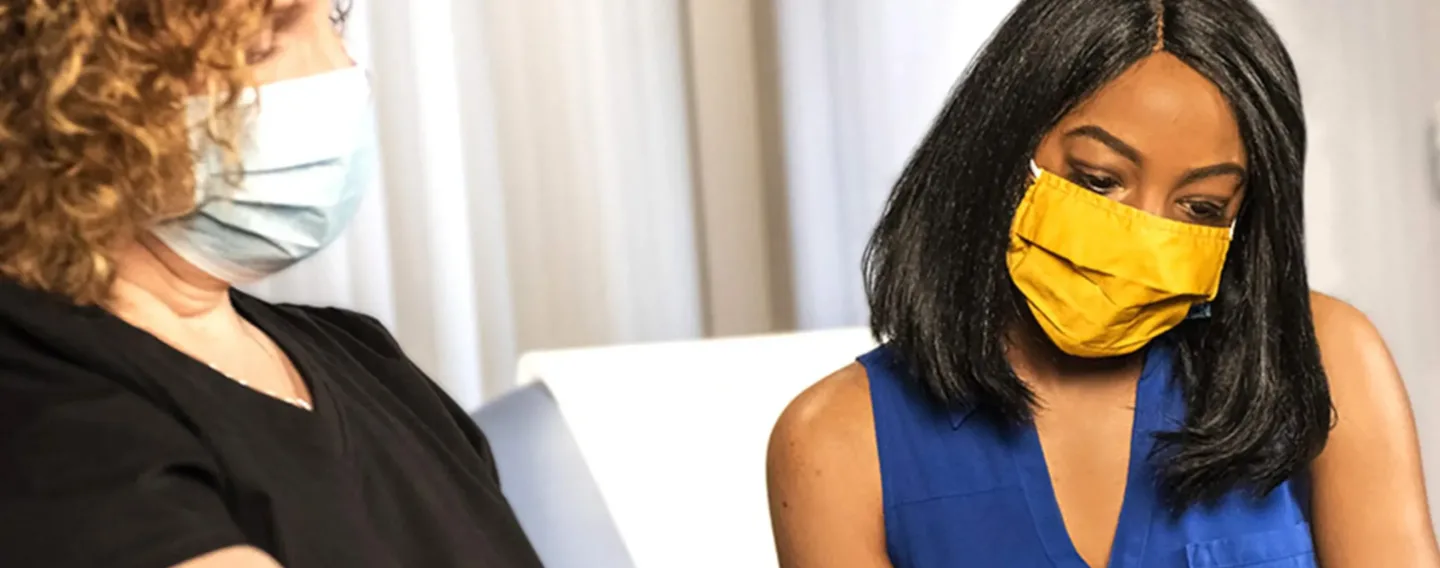