Medidata Blog
KRI Trending Graphs and Ending the Groundhog Day Loop
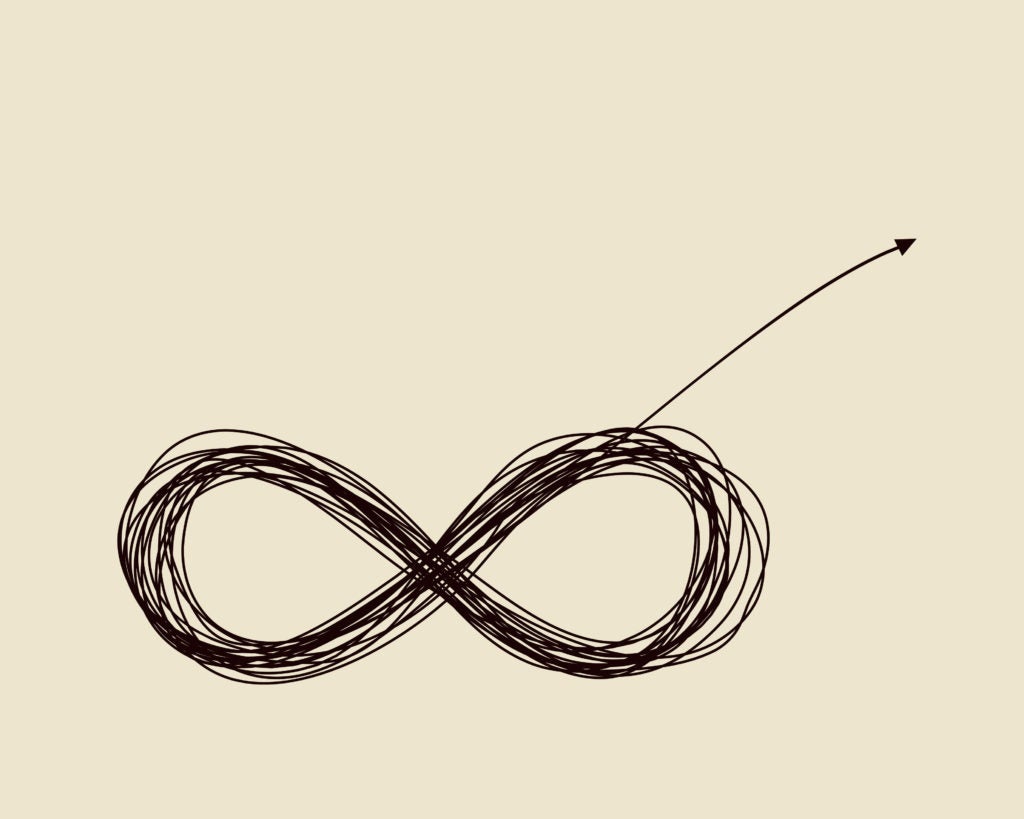
The famous quote “Those who fail to learn from history are doomed to repeat it” seems appropriate when describing the pharmaceutical industry, at least according to our track record over the last few decades. It often feels like Groundhog Day, and we are Bill Murray hitting the alarm clock before realizing “Oh, here we go again!”
The good news is that things are rapidly changing as the industry starts to leverage advanced analytics and the data assets that have been amassed in recent years. With these advancements, clinical teams now have the ability to learn where they are underperforming in real time and forge a new, brighter future. This is the vision behind risk-based monitoring and why the regulatory agencies are advocating the use of detailed risk management plans and centralized statistical methodologies. We simply need to work smarter to avoid repeating the same mistakes.
In order to create this learning process, technologies must deploy better visualizations that provide clinical researchers effective ways to view this history, so that they can constantly monitor where they have been, what they have tried, what has worked, and what has not. Probably one of the most effective visualizations is the Key Risk Indicator (KRI) trending graph. However, a KRI trending graph can’t simply be a tracing of the KRI level over time, but must include:
- An overlay of historical actions to determine what has been tried and what actions led to positive site improvement
- A sliding time scale allowing clinical teams to focus their evaluation on the most relevant data for any KRI
- Both site and study level trend lines so that site performance is viewed in context of how all sites are performing in aggregate
Probably the most common examples outside our industry are the financial market trending graphs that track constant market fluctuations and corporate performance. Anyone invested in the stock market typically starts by looking at the company’s stock performance trending graph. They look at the trend line for the most recent performance, but they also want to understand how the company has performed over the long run.
Sometimes companies experience a positive market uptick based on a specific recent event, but its erratic performance over the last few years indicates the recent trend is less reliable. In addition, you need to look at the company’s performance in comparison to overall market performance (e.g., DJIA or S&P 500), because certain internal changes may simply be a result of market influences.
By viewing both trend lines, an investor can focus on periods where company performance has deviated from the overall trend. If a company is outperforming the market over an extended period of time, this is the best signal that this company is consistently hitting its financial targets.
This analogy easily transfers to a risk-based monitoring approach to clinical trials. By incorporating KRI trending graphs with the aforementioned functionality, clinical teams possess an effective visualization tool to assess site performance over time, with a comparison of overall study trends, to determine when corrective actions must be applied. With the ability to quickly review associated corrective actions, the monitor will be able to consider what actions have already been tried so that effective escalations can occur if the initial actions were not successful.
As we move this technology concept one step forward, we envision the ability to track specific types of actions and grade certain categories of actions as more effective. This effectiveness score can be calculated through additional “big data” algorithms that associate percentage change in any given KRI trend line with the actions that occurred most recently prior to the improvement. This machine learning approach will enable clinical teams to learn from the past and achieve a more effective outcome for the future.
The ability to deliver this level of technology and data visualization requires a complete end-to-end solution for risk-based monitoring. This is why Medidata has focused on the Platform-as-a-Service (PaaS) model. Our PaaS model for RBM incorporates the following modules:
- Risk Management & Planning (RACT/IQRMP)
- Centralized Statistical Analytics (KRIs plus advanced machine-learning algorithms)
- Issue Management (study and site level corrective actions, including alerts and notifications)
- Site Monitoring solution allows for adaptive monitoring based on risk
- Master data to facilitate data aggregation and data learning
With each of these modules working together in a PaaS model, we are creating a fully traceable risk management solution that will free our customers from the Groundhog Day time loop phenomenon, and wake us all up to a brand new era.