Challenges & Opportunities in Implementing Generative AI
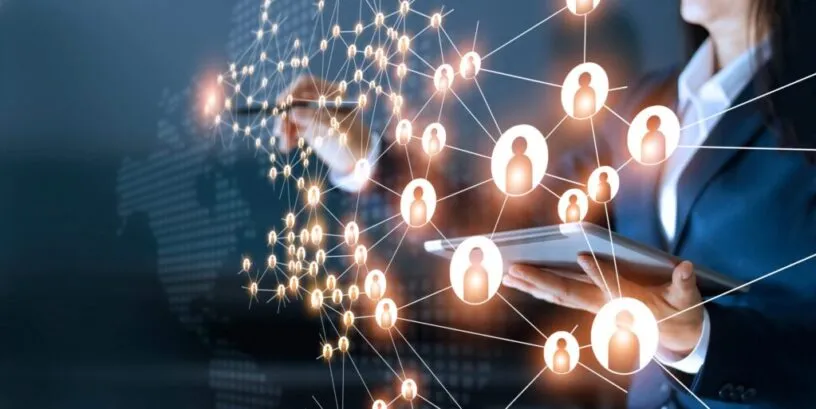
The potential that generative AI may have on nearly all industries is exciting. Though, like any new technological advancement, there are both challenges and opportunities to understand. For the life sciences industry, the stakes are particularly high due to the sensitive nature of the data and the growing adoption of this technology. Understanding these challenges and opportunities is vital for life sciences organizations navigating this evolving landscape.
Key Challenges in Implementing Generative AI
Some of the most prominent challenges in implementing generative (gen) AI technologies into the clinical trial landscape include:
- Obstacles around sharing data, especially for protected health information (PHI). Despite the safeguards and privacy protections built-in to gen AI algorithms and their outputs, like synthetic datasets, patients and organizations remain skeptical of this technology—in part due to its novelty. Patients and clinical trial participants may remain reluctant to reveal relevant health information due to the fear of it getting leaked, or improperly used in an algorithm. Similarly, organizations may be hesitant to implement gen AI technologies because of the strong concern of inadvertently misusing sensitive patient data.
- Potential inaccuracies in outputs. Like any technology, Gen AI is not infallible. But these risks can be mitigated, which is particularly important within the context of clinical development where precision is critical. To overcome this challenge, it’s essential to foster collaboration between human expertise and gen AI capabilities, rather than solely relying on one or the other (Harvard Business Review, 2023).
- Ensuring underlying data is accurate, complete, and “trusted” is critical for generative AI models, especially in the pharmaceutical industry where patient-level data is sensitive and complex. Poor data quality can lead to flawed insights, jeopardizing critical processes like clinical trials. Gen AI solutions must address these challenges by leveraging anonymized, high-quality data that is capable of delivering accurate and actionable outputs, ensuring trust and reliability in AI-driven decision-making.
Promising Opportunities with Generative AI
Despite the existing challenges in implementing generative AI into healthcare and pharma settings, many promising opportunities have arisen already, such as:
- Improving protocol design, which is a generally time consuming and expensive process, and can be the source of accidental roadblocks early on in a clinical trial program. Novel insights powered by high-fidelity synthetic data generated from historical trial data can help anticipate and mitigate common issues early on, reducing protocol amendments, as well as other delays and costs.
- Augmenting data, while maintaining patient data privacy and safety. Gen AI enables the creation of data that can augment existing datasets or findings, allowing companies to gain insights that would have previously been impossible.
- Addressing data privacy concerns. While the hesitancy to share health data is not new to clinical trials and healthcare in general, generative AI offers a novel way to overcome this persistent challenge. Synthetic datasets, created using gen AI algorithms, can mitigate these privacy concerns because they ensure patient data is anonymous and unidentifiable, while maintaining the utility of the dataset to clinical developers looking to generate evidence.
Looking Ahead
Generative AI is still in its early stages of adoption, but its potential impact on healthcare and life sciences is undeniable. To harness its benefits, companies must:
- Invest in understanding both the challenges and opportunities.
- Educate stakeholders—from patients to executives—on the safeguards and value of generative AI.
- Implement collaborative approaches that combine AI capabilities with human expertise.
As generative AI matures, its role in revolutionizing clinical research and healthcare will only grow. The organizations that proactively navigate its complexities today will be best positioned to lead tomorrow.
Contact Us
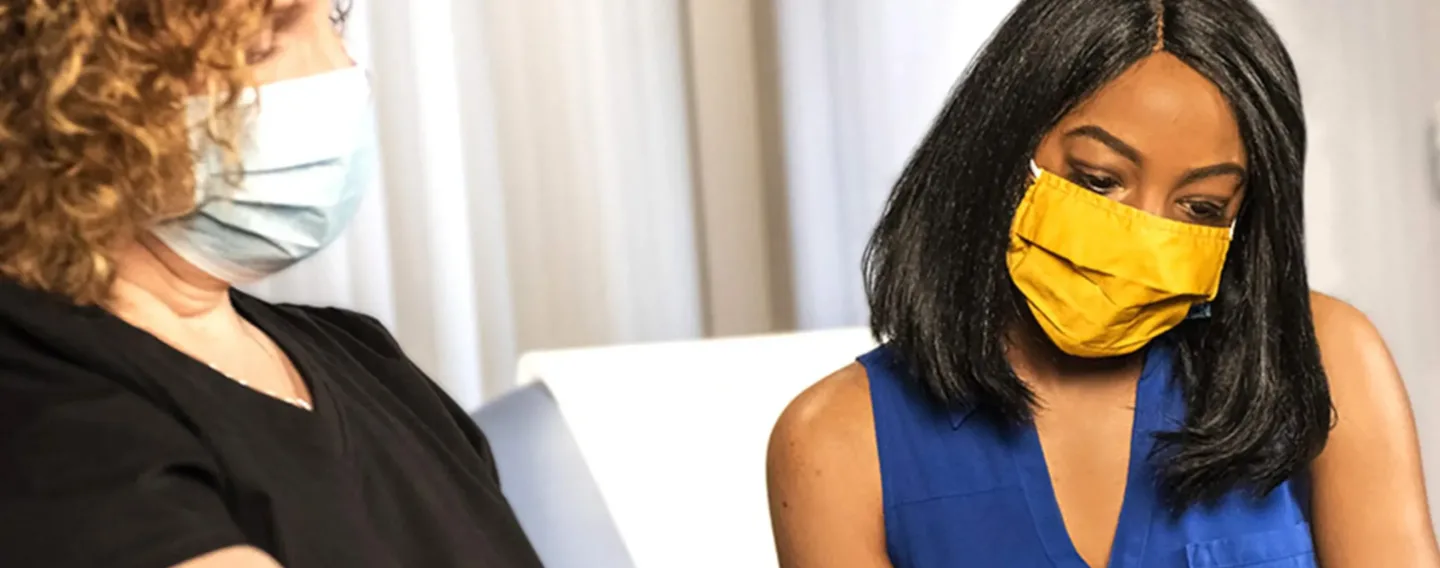