Medidata Blog
Demystifying AI-Powered Clinical Trial Data Quality: Unveiling the Mechanics
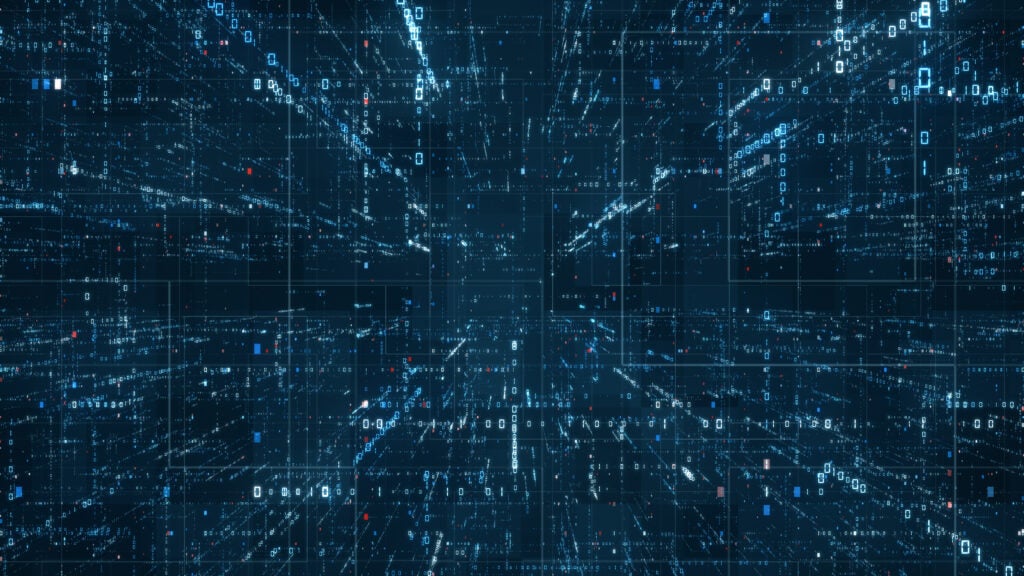
AI is a technology solution that addresses real and potentially crippling clinical trial challenges. It’s also unusual in that it stands apart from other technologies due to the mystique surrounding it. This may stem from preconceptions based on popular fiction, or the fact that AI technology is a departure from the rigid and limited logic of other systems—such as those based on ‘if-else’ statements. Or it could be due to the hype about this technology, which can impact first impressions of its validity.
While incapable of thought, AI systems and tools transform clinical research stakeholders across the entire ecosystem. But how does AI make a difference—and is it as good as it’s reported to be?
These questions necessitate deep answers within each area of the clinical trial process. But at a high level, we can use case studies to provide a picture of how AI transforms trials.
As clinical data management is critical to studies, we’ll highlight a real-world use case of why AI was introduced to a study and how that decision made such a difference.
To add context to this use case, due to the many benefits of complex clinical trial methodologies, there was an ever-increasing, rapid adoption rate of this method. One of the clear advantages and reasons for adopting complex studies is the depth and breadth of additional data collected. This has also meant that data complexity, increased data volumes, and the speed at which data is created have caused challenges in data management, reconciliation, aggregation, and quality management. Inconsistent data interoperability between multiple systems has also introduced further challenges.
Use Case: Identifying Missing Concomitant Medications Related to Adverse Events
Clinical trials involve extensive documentation of adverse events (AEs), patient comments, procedures, and medical histories. A critical issue in studies is the potential omission of adverse events or unreported medical issues. This is a highly complex, multi-faceted data management process that can be error-prone due to the use of disparate tools and high volumes of data from multiple sources—including forms from patients—that need to be processed before being presented to data managers for analysis and next steps.
The specific challenge in this use case was the sponsor’s need to identify missing concomitant medications (CMs) related to AEs. The aim was to make sure that all CMs were reported and that any AE requiring a CM but lacking one was red-flagged. Where the volume of data is very high, the aggregation, standardization, review, and reconciliation process was error-prone and slow—causing problems for data managers performing analysis that would lead to subsequent delays.
The AI-powered Solution—A Knowledge-Based Architecture for Expert Systems
The approach to solving this problem involved a knowledge-based architecture for expert systems. The key to this lies in mimicking the decision-making process of an expert, where the process begins with understanding the problem and leveraging rule-based approaches to identify relationships between adverse events and comments. But the real magic is found in the knowledge graph—a structured representation of the relationships between medications and conditions.
The knowledge graph was constructed by aggregating data from clinical trial records, third-party biomedical data, and historical trials. But creating a robust graph involves addressing the challenge of interoperability. Medications, for example, may be represented in various ways, necessitating a semantic component and ontologies for accurate identification.
To enhance the reliability of the knowledge graph, a machine learning model is employed to assign strengths to the edges of the graph, representing relationships between medications and the treatment of specific events.
After its implementation, while the sponsor had high expectations already, they were surprised by how powerfully and quickly the AI system could aggregate and standardize their data, one of their key pain points, making the review and reconciliation process straightforward and subsequently presenting data managers with clean data for analysis and action on time. The AI system's decision model (based on the ML model specifying the strength of edges) came into play during record linkage, where it determined the confidence level of identified missing CMs for specific AEs. The user was presented with results for adjudication—our human-in-the-loop process that ensures continuous improvement by the system, with user decisions contributing to the refinement of the knowledge graph and algorithms.
In Conclusion
Medidata’s AI reconciliation in clinical data management is a powerful tool, effectively addressing challenges in identifying missing information in adverse event forms, aided by AI-powered complex data aggregation, standardization, reconciliation, review, and analysis. By combining rule-based approaches, knowledge graphs, and machine learning, this approach showcases a holistic solution to a complex problem.
As technology advances, the marriage of AI and clinical research promises to revolutionize clinical trial data management.
Learn more about how Medidata is at the forefront of AI in Clinical Trials.