Medidata Blog
AI in Clinical Data Management: What Does It Do, and How Does It Work?
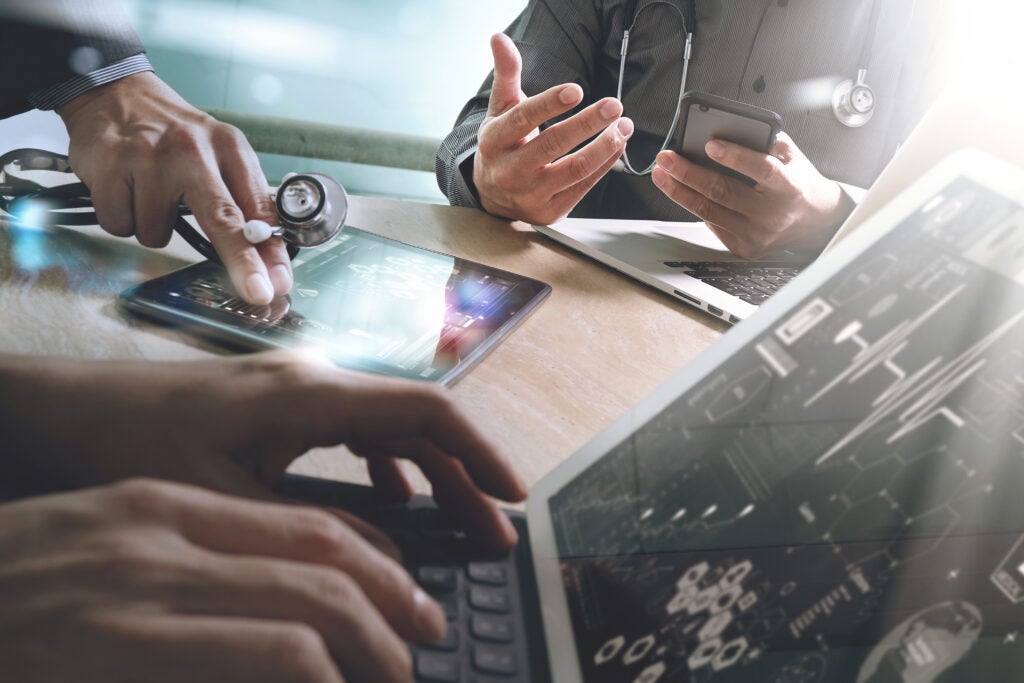
AI (artificial intelligence) addresses real clinical trial challenges and problems, significantly empowering data managers and other stakeholders. While AI brings transformational benefits, it also stands apart from other technologies, being shrouded in a certain level of mystery that divides opinion and can be a barrier to adoption. This is often due to preconceptions, misunderstandings, a lack of exposure to the AI tools that are available, and the absence of detailed information about how it works.
Here, we take a closer look at what AI and machine learning (ML) can do to help clinical data management in medical coding, data reconciliation, and audit trail reviews.
AI-assisted Medical Coding
During a clinical trial, descriptions of symptoms, procedures, medication related to adverse events (AE), medical history, and concomitant medications (CMs) are known as verbatims. Once verbatim terms are recorded, sponsors are required by the FDA and other regulatory authorities to code them to industry-standard dictionaries.
Examples of the MedDRA (Medical Dictionary for Regulatory Activities) dictionary, used for coding adverse events and medical history, and the WHODrug (World Health Organization Drug) dictionary for concomitant medications are shown in Figure 1.
A clinician may have recorded a patient’s AE as “headache”, which, depending on other information about the patient or study, might be coded to “throbbing headache” with a code of ‘10058140’ using the MedDRA dictionary. Another patient could be taking ‘paracetamol’, which would be coded as ‘000200’ using the WHODrug dictionary.
Figure 1. MedDRA and WHODrug example data.
During a study, medical coders have to code thousands of terms and have often spent many years building their expertise, creating proprietary synonym lists that are used and maintained to speed up the complex and time-consuming task of coding. When they’re presented with a powerful tool that can transform their work, the idea is appealing, and questions are raised about the tool’s capabilities, accuracy, and efficiency.
ML algorithms remove the need for coding specialists to carry out synonym maintenance and save significant time and effort on manual ‘browse & code’ processes.
As a foundation, Medidata’s predictive coding ML algorithm is trained and tested on over 60M historic coding decisions made by professional medical coders for verbatim drug, symptom, and procedure terms—30M+ for MedDRA and 30M+ for WHODrug, across multiple dictionary versions from thousands of studies.
When the algorithm is presented with a verbatim term it predicts a dictionary code the term should be coded to. The medical coder is provided with a confidence level in that prediction—high, medium, or low—replacing synonyms or time-consuming dictionary searches as the first means of coding a verbatim. The coder can then choose to code the term to the prediction (giving the algorithm a thumbs-up), or adjudicate the prediction (giving it a thumbs-down). Terms can be set to ‘autocode’ if a configured confidence threshold is reached, further speeding up the coding process.
Development of Predictive Models
Before historical coding decisions are used to build models, data is cleaned—verbatims and medical codes are standardized, augmented with content directly from the dictionary, and decommissioned codes are removed.
The dataset is split into training and test sets according to a time-based method: the training set uses the oldest portion of the overall dataset, while the test set uses the newest portion of the data. The training set is used to build the predictive models, while the test set evaluates the models’ performance.
As WHODrug and MedDRA codes are hierarchical, Medidata’s ML models are also hierarchical. A model is trained to predict the parent level of the code—PT (preferred term) in MedDRA or DRN (Drug Record Number in WHODrug)—and lower-level models for each unique parent code. Models are only trained on the verbatim text, so they consistently return the same code prediction, regardless of the user or study that the verbatim originated from.
Models are regularly “refreshed” with the latest data so that they are built using the most recent coding decisions and can make predictions for new dictionary releases.
AI-Assisted Medical Coding - Accuracy and Efficiency
Predictive coding saves time spent browsing and searching to code verbatims, but how accurate is it?
If a user selects the high-confidence threshold, MedDRA predictions are expected to be 96% accurate when compared to decisions made by experienced coders, and WHODrug predictions are expected to be 92% accurate. The difference in accuracy levels results from the fact that MedDRA predictions result in the selection of a single code, whereas WHODrug predictions—though the AI predicts the drug code—require the user to select the appropriate ATC (anatomical therapeutic chemical) code.
The expected accuracy for medium and low confidence levels is slightly less, but results in an increase in the number of verbatims that can be autocoded.
A manual dictionary ‘browse and search’ process takes an average of five minutes to code each verbatim, compared to a few seconds through AI predictions. As studies may have thousands of verbatims to code, AI autocoding can save tens or even hundreds of hours per clinical trial. A high confidence level threshold for autocoding saves as much as 69 hours for every 1,000 verbatims autocoded. Because predictions are generated for every verbatim—even for terms that are not autocoded—users have reported significant time savings from prediction-assisted manual coding. Downstream benefits include coded term availability for data review, and the delivery of already-coded terms to the safety system, saving duplicate effort.
AI for Data Reconciliation
Reconciling data from different datasets is a perfect use case to highlight AI’s role as an ideal virtual assistant that automates manual detailed tasks and drives process efficiency and innovation.
An AI expert system trained with a knowledge-based architecture can assess and rank complex associations between adverse events (AEs), concomitant medications (CMs), and medical history datasets and assign confidence levels for those associations using historical clinical trial data, open source models, and dictionaries.
Because AI data reconciliation is algorithmically powered, users will save hours formerly devoted to time-intensive manual listing reviews and de-risk data management by reducing the oversight needed for complex data quality checks. Items that may have otherwise been missed are more likely to be flagged by the AI and automation.
When, for example, a report to find AEs without CMs is run, the system references the knowledge graph of ranked associations between AEs and CMs, identifies potential discrepancies between the datasets, and suggests associations that need to be created to fix them. The report lists the suggestions for the user to review and link using the same thumb-up/down adjudication process described above for medical coding. This human-in-the-loop adjudication process feeds and improves the expert system, too.
With AI-assisted data reconciliation, data managers no longer need to manually review multiple listings to try to find discrepancies.
Audit Trail Review (ATR)
Clinical trial audit trails are vast, containing every aspect of a study—including clinical data, queries, system logs, activity logs, metadata, and other information. Typically, these trails span multiple systems and processes, so querying them for an ATR is challenging. Even with centralized audit trail data, analysis can be burdensome, and responses to queries from regulators can take significant time and effort.
Medidata is developing a capability that leverages generative AI to analyze audit trail logs, making it easier to understand and report on trends, see the context of data changes, and view the sequence of events. The user experience is simplified by smart prompts and embedded chat to generate ATR results. The system establishes data integrity controls throughout the data lifecycle, giving stakeholders confidence and trust in the integrity, accuracy, transparency, and quality of the ATR. Regulators’ questions will receive faster responses to requests.
With generative AI, accuracy depends on having the right unbiased data and the use of appropriate prompts to query the system.
Overcoming Fears and Barriers to Adoption
Transformation through AI, once embraced, takes clinical trials to a whole new level.
Adopters have found that they can face hesitation from some stakeholders—there may be concerns about how their roles will evolve or which roles could be combined or replaced by AI. These reactions may be based on conjecture, preconceptions, or misunderstandings, and the way to move forward is to empower stakeholders with a clear understanding of AI/ML—what it does, how it works, and how it can positively transform user focus and workloads, clinical trials, and the lives of patients.
Our recommendation to achieve this is to build a foundation of clarity and understanding through a four-pillar upskilling approach:
- Increase Literacy
To increase literacy in and understanding of AI, provide teams with the following:
- AI learning programs and a knowledge base infrastructure
- Interactive learning with examples
- An understanding of the iterative nature of AI
- A way to identify new business cases and support evolution
- Put Clinical Data Managers in the Loop
To make sure that clinical data management teams feel like they’re in control of how AI is used, ensure:
- Feedback expectations are clear
- The impact of the AI feedback loop is understood
- Advantages and shortcomings are understood (e.g., insufficient or low quality of data and bias)
- Manage Change
To achieve the successful adoption of AI, focus on:
- Changing mindsets
- Eliminating fears of the unknown and job losses
- Increasing comfort levels through training
- Building confidence by selecting champions
- Validation
To reduce the potential bias in AI:
- Emphasize the need for human-in-the-loop adjudication
- Test across multiple subsets of data
- Perform sensitivity tests
- Use real-world evidence for validation
- Work with regulators
In Conclusion
The examples we’ve touched on show how AI helps clinical data managers by automating time-consuming, complex data management processes in medical coding, data reconciliation, and ATRs. This lets them use their skills, experience, and knowledge to focus on ensuring the delivery of high-quality data for analysis.
The same principle applies across the entire clinical trial landscape, where AI can transform the way people work by handling the most complex, time-intensive tasks, and, in turn, take the evolution of clinical trials to a completely new level.
Although it’s widely acknowledged that the impact of AI in the clinical trials industry is and will continue to be transformational, it’s an often misunderstood technology that can nurture doubt, concern, and even fear. To help everyone take advantage of the power of AI, transparency, inclusion, education, and hands-on experience are the cornerstones of successful adoption.
This will empower stakeholders with the ability to transform their roles, their clinical trials, and the lives of patients while adding very relevant and differentiating skill sets.
AI—it’s everybody’s business.
Read the white paper ‘Accelerate Precise Medical Coding in Clinical Trials’ to learn more about the AI coding algorithm used in Rave Coder+.
See how Medidata Clinical Data Studio is leveraging AI, automation, and smart analytics to deliver a complete data management and quality experience that integrates and analyzes multi-source data to shorten review timelines and improve data reliability.